Author: Gershon Ben Keren
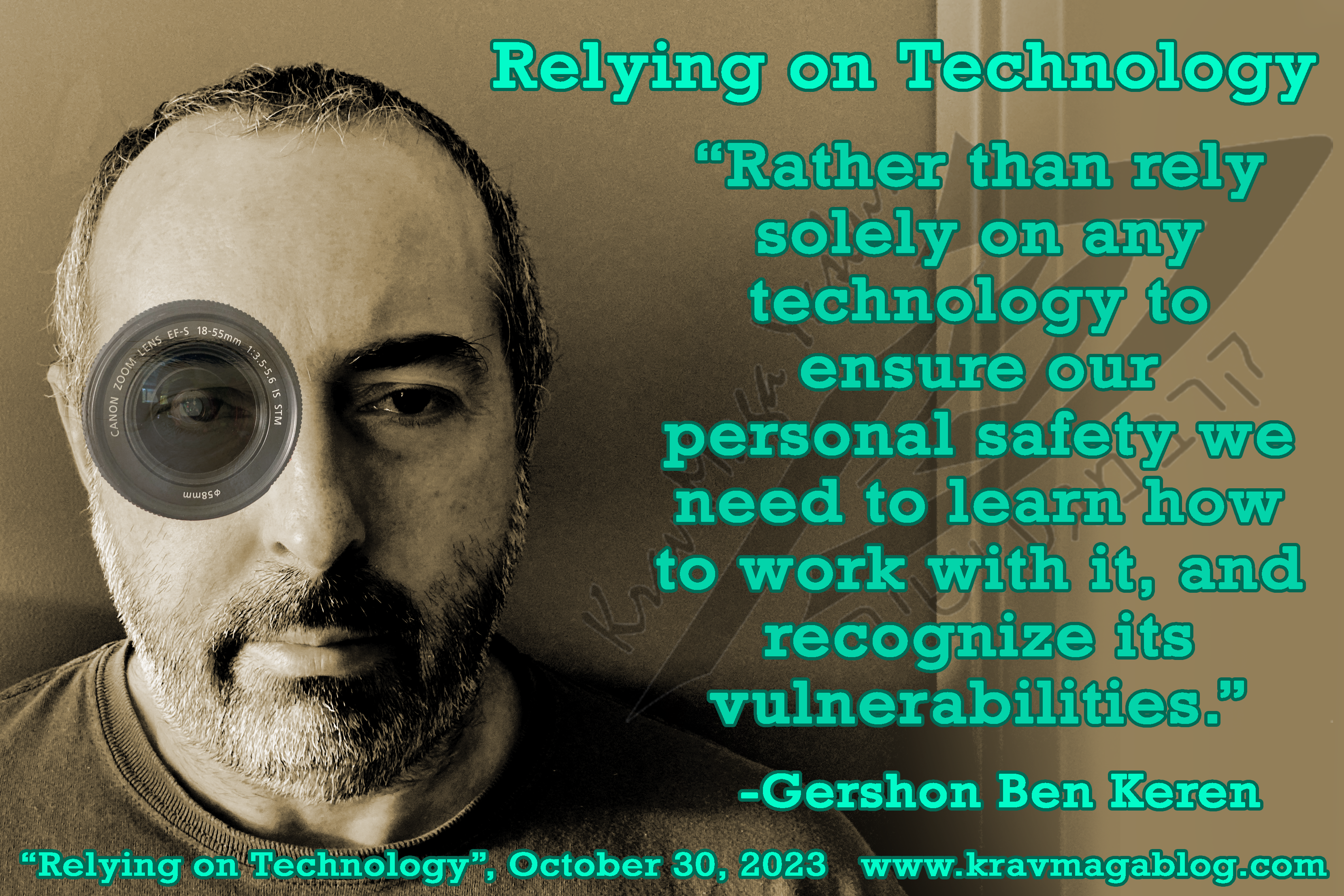
I am a fan of AI (Artificial Intelligence). I first started using machine learning to analyze data sets somewhere around five or six years ago and found that the machine does it much better than me. My manual linear regression analysis was significantly inferior to my computer’s multi-linear regression analysis, which it did in a fraction of the time. I didn’t instantly accept this, but over time came to find that the computer could analyze data, consistently, in many more different ways than I could, and come out with more useful results e.g., the computer was better at using past crime data for forecasting where and when future crime would take place etc. I’m not saying that any of this analysis is “new” or “revolutionary” but that I’m a fan of using technology for crime detection and prevention. I was fortunate enough to do some work with the CCTV (Closed Circuit TV) operators who assisted transit police in the UK, which was a great example of trained individuals using technology to identify offenders who were about to offend e.g., they had come to recognize individuals who didn’t look up to the displays which told when the next train was coming (something everyone actually riding the transit system did), and “passengers” who would move between northbound and southbound platforms, without getting on a train etc. These operators could watch multiple stations and locations in a way that someone on the ground couldn’t. It was a great partnership between humans and technology, however from an efficiency point of view the more the machine and/or camera could do and take the burden of the operator, the more locations and stations could be monitored. In this article I want to look at the effectiveness of recognition technologies both for faces and car registration/number plates.
Early facial recognition technology that began in the 1960’s and 1970’s relied mainly upon manual measurements of facial features. In the 1980’s this method was largely replaced by eigenfaces, which basically involved plotting faces as vectors, with a pixel or group of pixels containing data/information about that particular part of the image, on which lines and axes are drawn (eigenvectors) where the data varies the most. To recognize a face, an image of a face is projected onto this, to see how closely its eigenvectors match. One of the restrictions this method had was that it reduced the face to a two-dimensional model, and so could only have a chance at being accurate if the face to be recognized was captured directly front-on – in fact, most of the software developed to do such facial recognition at the time stated this. From a security perspective this was an extreme restriction because facial recognition would only work if someone looked directly at a camera. In the early 2000’s 3-D facial recognition became available, which was greatly enhanced in the last decade (2010’s) as AI, neural networks, and machine learning came to play a role. However, despite these quite amazing technological advances, facial recognition is far from perfect/accurate. One of the issues that any machine learning software has is the sample of data it learns from (supervised machine learning), and how strict the parameters are for how it learns. If the sample dataset that it uses to learn from contains only white, male faces, the “machine” will get good at learning how to identify white male faces, but in applying the “rules” it has learnt to women, people belonging to other ethnic groups etc., its “predictions” (how closely someone’s face matches a stored image), may be highly inaccurate. Other factors also come into play to confound the problem. If a stored image in a database was taken under a certain light setting, such as a traditional police “mugshot” using flash photography, this will likely lighten the skin tone of a person. If a CCTV image taken late at night is compared to this, without accounting for the change in light, then a match is unlikely to be made, as without good light the skin color will likely appear much darker i.e., comparing images in controlled and uncontrolled environments is more likely to lead to inaccuracies. Also, the near impossibility of keeping up to date stored images of faces is going to mean that aging will make FR (facial recognition) a more complex task. This is not to say that FR doesn’t benefit the CCTV operator, as a computer is far faster at processing the faces in a crowd than a human. If FR can help filter and reduce the number of persons of interest to be investigated/studied, then this “assistance” will aid an operator greatly.
Whilst facial features can vary significantly, numbers and letters don’t. Especially if the same font/typeset is used. The first “fixed” speed camera installed in the UK, was in 1992, in Twickenham, and was calibrated to catch only the very worst offenders (in the UK these cameras are known as a “Gatso” after Maurice Gatsonides, a Dutch rally driver and inventor, who used cameras to measure his driving speed). Modern day “fixed” cameras claim they are calibrated to a 2% degree of accuracy. This figure is lower for mobile cameras that may be set up temporarily on stretches of road. Whilst these UK cameras are primarily designed to capture offenders who speed, they also catch stolen and unlicensed vehicles, and those who are driving unlicensed and uninsured (around 17% of all captures). One of the big civil criticisms is that jurisdictions both in the UK and US use them more as a means to generate revenue than enforce safety, however as someone who was once hit by an uninsured driver and suffered the economic consequences of this (at a time when I was young and really couldn’t afford the costs of repair), the more uninsured drivers that can be identified and forced into getting insurance, the better. In a two-year (2013-2015) period Chicago erroneously issued $2.4 million in fines e.g., in areas where there wasn’t correct speed limit signage, and at times when a particular speed limit shouldn’t have been enforced (in school areas when schools were on vacation). In the U.S., there have also been issues where it was impossible to identify which car tripped a camera – often the person making the decision will simply default to the one nearest the camera, without any other evidence. However, wherever technology exists to do one thing, technology exists to block it e.g., “noPhoto” devices exist to create a light flash, which obscures the number/registration plate where red-light speed cameras are detected, and then there are laser jammers that intercept police lasers, and then bounce them back at the same frequency etc. There are also companies that will provide “cloned” plates that match the year and model of your car, but in fact belong to someone else’s similar car that may be located in another city/state/locale etc. A ”trick” that some New Yorkers are using is rather than use another car’s number/registration plate is to put a plastic film/sheet over theirs which pixelates the plate e.g., presents the number three as a “B” by drawing the requisite lines etc.
Whenever technology is used to either enforce a law, or even prevent a crime, offenders find a way to circumvent or exploit it. Self-driving cars are overall statistically safer than human drivers but they’re not perfect – which is why we have concerns about them. Facial Recognition (FR) software/programs are extremely effective, as are speed cameras and their like, but they’re not perfect and they either have failings and/or people find ways to exploit their vulnerabilities etc. Rather than rely solely on any technology to ensure our personal safety we need to learn how to work with it, and recognize its vulnerabilities.